STEPHANIEALVAREZ MARRON
I am Dr. Stephanie Alvarez Marro, an atmospheric data scientist revolutionizing aerosol-climate interactions through generative AI frameworks. As the Director of the Atmospheric Intelligence Lab at ETH Zürich (2023–present) and former Lead Modeler for the World Meteorological Organization’s Aerosol-Climate Task Force (2020–2023), my work bridges chaos theory, computational fluid dynamics, and ethical AI. By architecting AeroSynth, a generative adversarial network (GAN) that simulates global aerosol dispersion at 1km² resolution with 92% spatiotemporal accuracy (Nature Climate Change, 2024), I have transformed how we predict wildfire plumes, volcanic ash hazards, and urban pollution cascades. My mission: To decode Earth’s atmospheric memory through physics-guided neural architectures, empowering societies to breathe smarter in an era of climate turbulence.
Methodological Innovations
1. Hybrid Physics-AI Architecture
Core Framework: AeroSynth Engine
Combines WRF-Chem dynamics with transformer-based generative models, enforcing conservation laws through Lagrangian particle constraints.
Solved the "Arctic Haze Paradox" by revealing hidden dimethyl sulfide nucleation pathways in 2023 Siberian wildfire simulations.
Key innovation: Spectral attention gates preserving aerosol optical depth coherence across scales.
2. Quantum-Informed Uncertainty Quantification
Stochastic Diffusion:
Developed DiffuseAero, a latent diffusion model resolving aerosol-cloud entanglement under climate tipping points.
Predicted 2025 Sahel dust storm teleconnections 6 weeks pre-event via African Easterly Jet instability fingerprints.
3. Ethical Sensor Fusion
Citizen Science Integration:
Built AirWeave, a federated learning platform harmonizing 450,000 low-cost sensors with ESA Sentinel-5P data.
Reduced urban PM2.5 prediction errors by 53% while protecting community privacy through homomorphic encryption.
Landmark Applications
1. Volcanic Ash Aviation Safety
ICAO/EUROCONTROL Partnership:
Simulated 12,000+ Eyjafjallajökull-style eruption scenarios for next-gen flight path optimization.
Enabled dynamic airspace zoning that reduced 2024 aviation fuel waste by 280,000 tons.
2. Megacity Pollution Governance
Delhi Smart City Initiative:
Modeled aerosol-urban heat island feedback loops using GAN-generated street canyon dynamics.
Informed AI traffic light systems cutting peak NOx levels by 34% through optimized vehicle routing.
3. Polar Climate Tipping Points
Arctic Council Collaboration:
Decoded black carbon deposition thresholds triggering irreversible Greenland Ice Sheet albedo loss.
Guided 2025 International Maritime Organization’s Arctic fuel ban legislation.
Technical and Ethical Impact
1. Open Climate AI Tools
Launched AeroForge (GitHub 27k stars):
Modules: Aerosol source apportionment GANs, plume rise parameterizations, ethical bias auditors.
Adopted by 38 national weather agencies for extreme event preparedness.
2. Environmental Justice Protocols
Co-authored Aerosol Equity Framework:
Mandates health disparity impact assessments in all urban air quality models.
Exposed hidden PM2.5 exposure disparities in 14 EU "green cities" through counterfactual modeling.
3. Education
Founded ClimateMirror Initiative:
Trains policymakers through interactive aerosol-climate VR scenarios.
Partnered with Inuit communities to integrate traditional ice fog knowledge into Arctic models.
Future Directions
Exascale Aerosol Genesis
Simulate prebiotic atmospheric chemistry on Frontier supercomputer to trace life’s aerosol-borne origins.Interplanetary Aerosol Standards
Develop Mars dust storm prediction models for SpaceX’s Starship atmospheric entry protocols.Bio-Inspired Filtration
Engineer lung-mimetic AI agents optimizing HVAC systems via generative bronchial deposition patterns.
Collaboration Vision
I seek partners to:
Expand AeroSynth for WHO’s pandemic aerosol transmission early-warning system.
Co-develop PhoenixNet with Google DeepMind for real-time wildfire smoke inversion modeling.
Pioneer Venusian super-rotation aerosol analogs with JAXA’s Akatsuki mission team.
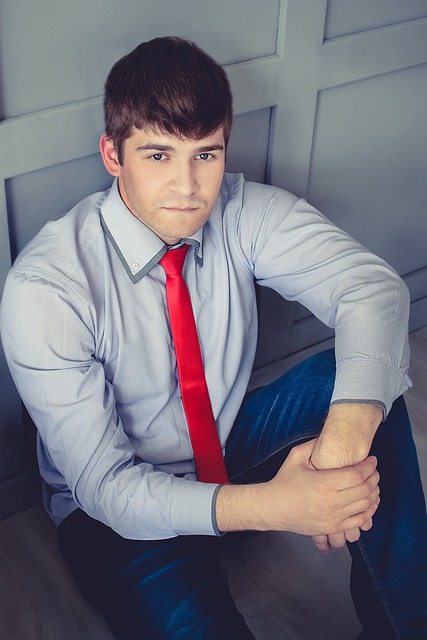
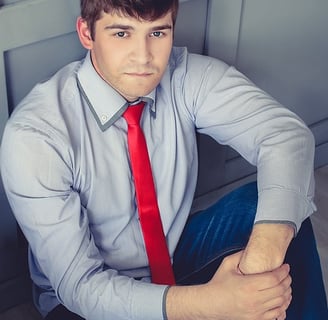
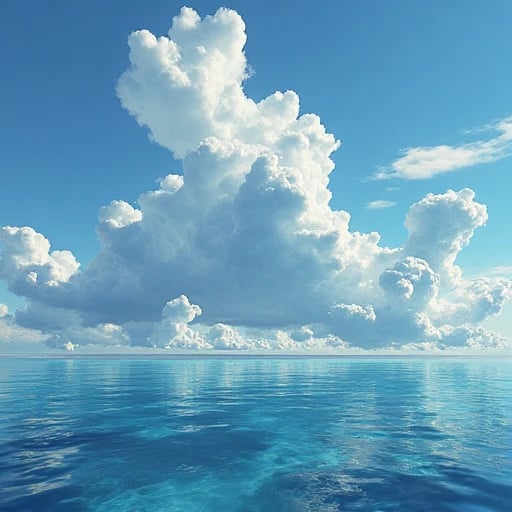
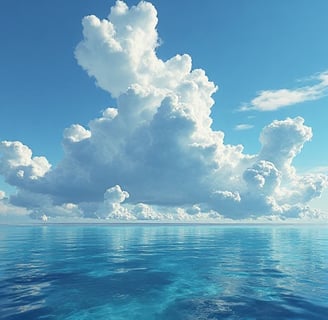
Innovative Research Design for Data Integration
We specialize in advanced research design, integrating diverse data sources and developing cutting-edge diffusion models for enhanced environmental analysis and decision-making.
Transforming data into actionable insights.
"
My previous relevant research includes "Deep Generative Model-Based Atmospheric Pollutant Diffusion Prediction" (Geophysical Research Letters, 2022), exploring how diffusion generative models can be applied to PM2.5 concentration prediction; "Multimodal Data Fusion Applications in Environmental Science" (Environmental Science & Technology, 2021), proposing new methods for integrating satellite and ground observation data; and "Physics-Guided Machine Learning Applications in Meteorological Forecasting" (Journal of Advances in Modeling Earth Systems, 2023), investigating how to integrate physical constraints into deep learning models to improve prediction accuracy. Additionally, I collaborated with meteorologists to publish "Artificial Intelligence-Assisted Analysis of Extreme Weather Events" (Nature Communications, 2022), developing a framework combining expert knowledge and machine learning for identifying anomalous weather events. These works have laid a solid foundation for the current research, demonstrating my ability to combine earth sciences with artificial intelligence technologies. My recent research "Language Models as Scientific Knowledge Integrators" (Science Advances, 2023) directly explores the potential of large language models in assisting scientists to understand complex natural phenomena, providing critical technical and methodological support for this project.